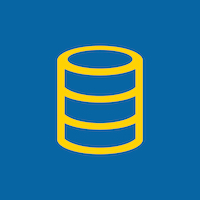
Human Activity Recognition Using Smartphones
Donated on 12/9/2012
Human Activity Recognition database built from the recordings of 30 subjects performing activities of daily living (ADL) while carrying a waist-mounted smartphone with embedded inertial sensors.
Dataset Characteristics
Multivariate, Time-Series
Subject Area
Computer Science
Associated Tasks
Classification, Clustering
Feature Type
-
# Instances
10299
# Features
-
Dataset Information
Additional Information
The experiments have been carried out with a group of 30 volunteers within an age bracket of 19-48 years. Each person performed six activities (WALKING, WALKING_UPSTAIRS, WALKING_DOWNSTAIRS, SITTING, STANDING, LAYING) wearing a smartphone (Samsung Galaxy S II) on the waist. Using its embedded accelerometer and gyroscope, we captured 3-axial linear acceleration and 3-axial angular velocity at a constant rate of 50Hz. The experiments have been video-recorded to label the data manually. The obtained dataset has been randomly partitioned into two sets, where 70% of the volunteers was selected for generating the training data and 30% the test data. The sensor signals (accelerometer and gyroscope) were pre-processed by applying noise filters and then sampled in fixed-width sliding windows of 2.56 sec and 50% overlap (128 readings/window). The sensor acceleration signal, which has gravitational and body motion components, was separated using a Butterworth low-pass filter into body acceleration and gravity. The gravitational force is assumed to have only low frequency components, therefore a filter with 0.3 Hz cutoff frequency was used. From each window, a vector of features was obtained by calculating variables from the time and frequency domain. Check the README.txt file for further details about this dataset. A video of the experiment including an example of the 6 recorded activities with one of the participants can be seen in the following link: http://www.youtube.com/watch?v=XOEN9W05_4A An updated version of this dataset can be found at http://archive.ics.uci.edu/ml/datasets/Smartphone-Based+Recognition+of+Human+Activities+and+Postural+Transitions. It includes labels of postural transitions between activities and also the full raw inertial signals instead of the ones pre-processed into windows.
Has Missing Values?
No
Introductory Paper
By D. Anguita, A. Ghio, L. Oneto, X. Parra, Jorge Luis Reyes-Ortiz. 2013
Published in The European Symposium on Artificial Neural Networks
Variables Table
Variable Name | Role | Type | Description | Units | Missing Values |
---|---|---|---|---|---|
no | |||||
no | |||||
no | |||||
no | |||||
no | |||||
no | |||||
no | |||||
no | |||||
no | |||||
no |
0 to 10 of 561
Additional Variable Information
For each record in the dataset it is provided: - Triaxial acceleration from the accelerometer (total acceleration) and the estimated body acceleration. - Triaxial Angular velocity from the gyroscope. - A 561-feature vector with time and frequency domain variables. - Its activity label. - An identifier of the subject who carried out the experiment.
Papers Citing this Dataset
By Yasin Yilmaz. 2017
Published in 2017 IEEE International Symposium on Information Theory (ISIT).
By Dewi Ismi, Shireen Panchoo, Murinto Murinto. 2016
Published in International Journal of Advances in Intelligent Informatics.
0 to 3 of 3
Reviews
There are no reviews for this dataset yet.
pip install ucimlrepo
from ucimlrepo import fetch_ucirepo # fetch dataset human_activity_recognition_using_smartphones = fetch_ucirepo(id=240) # data (as pandas dataframes) X = human_activity_recognition_using_smartphones.data.features y = human_activity_recognition_using_smartphones.data.targets # metadata print(human_activity_recognition_using_smartphones.metadata) # variable information print(human_activity_recognition_using_smartphones.variables)
Reyes-Ortiz,Jorge, Anguita,Davide, Ghio,Alessandro, Oneto,Luca, and Parra,Xavier. (2012). Human Activity Recognition Using Smartphones. UCI Machine Learning Repository. https://doi.org/10.24432/C54S4K.
@misc{misc_human_activity_recognition_using_smartphones_240, author = {Reyes-Ortiz,Jorge, Anguita,Davide, Ghio,Alessandro, Oneto,Luca, and Parra,Xavier}, title = {{Human Activity Recognition Using Smartphones}}, year = {2012}, howpublished = {UCI Machine Learning Repository}, note = {{DOI}: https://doi.org/10.24432/C54S4K} }
Creators
Jorge Reyes-Ortiz
Davide Anguita
Alessandro Ghio
Luca Oneto
Xavier Parra
DOI
License
This dataset is licensed under a Creative Commons Attribution 4.0 International (CC BY 4.0) license.
This allows for the sharing and adaptation of the datasets for any purpose, provided that the appropriate credit is given.